Faculty Profile
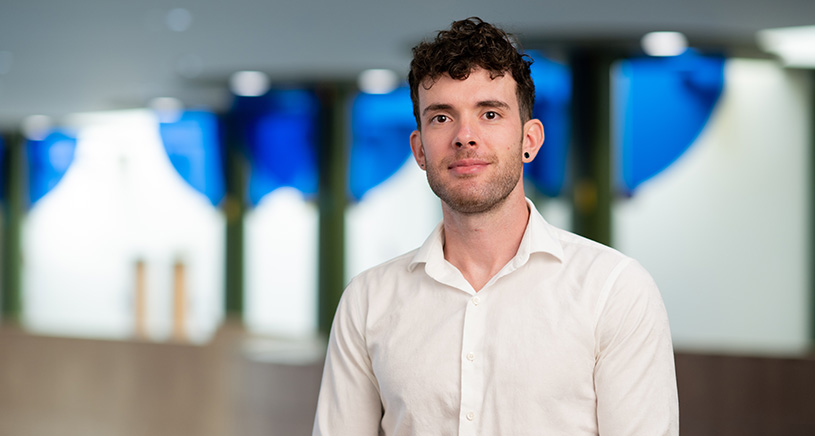
Michele Peruzzi, PhD
Assistant Professor, Biostatistics
I am interested in the development of Bayesian methods for multivariate correlated
data, with a specific focus on geostatistics for environmental health applications
as well as on imaging and high resolution sensor data. In addition to methods development,
I am active in building new software packages for reproducible and scalable statistical
analyses of large scale datasets.
- PhD, Statistics, Università Bocconi, 2019
- MSc, Economic and Social Sciences, Università Bocconi, 2013
- BSc, Economic and Social Sciences, Università Bocconi, 2010
My research interests include spatial statistics, Bayesian methods, models for complex
sensor data, environmental health, and climate change.
My current projects involve the development of scalable Bayesian methods for (1) analyzing complex, correlated, multivariate high resolution sensor data, including satellite imaging, air quality sensors, and medical imaging; (2) characterizing complex interaction effects in response surface models for large scale health data; (3) understanding the impacts of climate change on natural ecosystems and human health.
My current projects involve the development of scalable Bayesian methods for (1) analyzing complex, correlated, multivariate high resolution sensor data, including satellite imaging, air quality sensors, and medical imaging; (2) characterizing complex interaction effects in response surface models for large scale health data; (3) understanding the impacts of climate change on natural ecosystems and human health.
M. Peruzzi, S. Banerjee & A.O. Finley (2022), Highly Scalable Bayesian Geostatistical Modeling
via Meshed Gaussian Processes on Partitoned Domains. Journal of the American Statistical
Association 117(538): 969-982. https://www.tandfonline.com/doi/full/10.1080/01621459.2020.1833889
M. Peruzzi & D.B. Dunson (2022), Spatial Multivariate Trees for Big Data Bayesian Regression. Journal of Machine Learning Research 23(17):1−40. https://www.jmlr.org/papers/v23/20-1361.html
N. Neupane, M. Peruzzi, L. Ries, A. Arab, S. J. Mayor, J. C. Withey, A. O. Finley (2022) A novel model to accurately predict continental-scale green-up timing. International J. of Applied Earth Observation and Geoinformation 108:102747 https://doi.org/10.1016/j.jag.2022.102747
M. Peruzzi & D.B. Dunson (2022), Spatial Multivariate Trees for Big Data Bayesian Regression. Journal of Machine Learning Research 23(17):1−40. https://www.jmlr.org/papers/v23/20-1361.html
N. Neupane, M. Peruzzi, L. Ries, A. Arab, S. J. Mayor, J. C. Withey, A. O. Finley (2022) A novel model to accurately predict continental-scale green-up timing. International J. of Applied Earth Observation and Geoinformation 108:102747 https://doi.org/10.1016/j.jag.2022.102747
M4531 SPH II
1415 Washington Heights
Ann Arbor, MI 48109-2029
Email: peruzzi@umich.edu
Areas of Expertise: Biostatistics