Faculty Profile
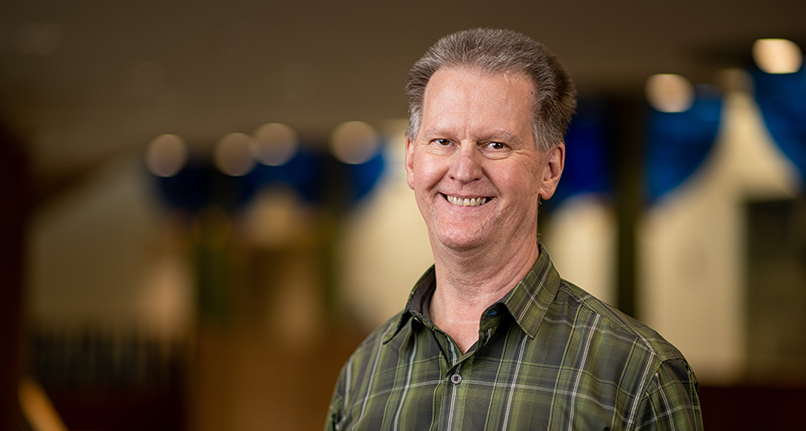
Timothy D Johnson, PhD
- Professor, Biostatistics
Statistical modeling of biomedical data. Bayesian and MCMC methodology with and emphasis on mixture models, highly correlated high dimensional data, and variable parameter spaces. Applications in medical imaging, neurology, cancer, radiology, and endocrinology.
- PhD, University of California, Los Angeles, 1997
- MS, University of California, Riverside, 1986
- BS, University of California, Riverside, 1984
- Bayesian methods and MCMC.
- Statistical image analysis.
- Spatial point processes.
- Statistical modeling of biomedical data.
- Applications in neuroscience, cancer, radiology, radiation oncolocy, Psychology/Psychiatry, endocrinology, and medical imaging.
Research Projects:
Bayesian methods for big imaging data. Along with Professor Jian Kang and graduate
students, we are developing fast Bayesian algorithms to handle massively large imaging
data sets such as those from the ABCD Consortium and the UK Biobank.
Bayesian methods for functional near-infrared spectroscopy (FNIRS). Along with Ioulia Kovelman, Assoc. Prof. of Psychology, I am developing fully Bayesian methods to analyze the spatio-temporal data obtained by fNIRS.
Bayesian methods for the analysis of pre-surgical fMRI data. I am interested in Bayesian method to analyze single subject fMRI data in the pre-surgical setting. Neurosurgeons are increasingly relying on fMRI data to assist in pre-surgical planning. What is unique and different about this setting is that false-negatives are arguably more important than false-positive signals and both types of errors need to be accounted.
Samartsidis, P., Eickhoof, C. R., Eickhoff, S. B., Wager, T. D., Feldman Barrett,
S., Atzil, S., Johnson, T. D., Nichols, T. E. (2018) Bayesian log-Gaussian Cox process regression: with applications
to meta-analysis of neuroimaging working memory studies. JRSSC
Teng, M., Johnson, T. D., Nathoo, F. S. (2018) Time Series Analysis of fMRI Data: Spatial Modelling and Bayesian
Computation. Statistics in Medicine 37 2753-2770. DOI: 10.1002/sim.7680
Liu, Z., Bartsch, A. J., Berrocal, V. J., Johnson, T. D. (2018) A mixed-effects, spatially varying coefficients model with application to
multi-resolution functional magnetic resonance imaging data. Statistical Methods in Medical Research DOI: 10.1177/0962280217752378
Montagna, S., Wager, T., Feldman Barrett, L., Johnson, T. D., Nichols, T. E. (2017) Spatial Bayesian Latent Factor Regression Modeling of Coordinate-based
Meta-analysis Data. Biometrics PMCID: PMC5682245.
Samartsidis, P., Montagna, S., Johnson, T. D., Nichols, T. E. (2017) The Coordinate-Based Meta- Analysis of Neuroimaging Data. Statistical Science 32(4) 580-599. DOI: 10.1214/17-STS624, PM- CID: PMC5849270.
Teng, M., Nathoo, F., Johnson, T. D. (2017) Bayesian Computation for Log-Gaussian Cox Pro- cesses: A Comparative Analysis of Methods. Journal of Statistical Computation and Simulation 87:11, 2227-2252, DOI: 10.1080/00949655.2017.1326117, PMCID: PMC5708893.
Liu, Z., Berrocal, V. J., Bartsch, A. J., Johnson, T. D. (2016) Pre-surgical fMRI data analysis using a spatially adaptive conditionally autoregressive model. Bayesian Analysis 11(2), 599-625. PMCID: PMC4814103.
Carlson, N. E., Grunwald, G. K., Johnson, T. D. (2016) Using Cox cluster processes to model the pulse generating mechanism driving time series of hormone data. Biostatistics 17(2), 320-333. doi:10.1093/biostatistics/kxv046.
M4065 SPH II
1415 Washington Heights
Ann Arbor, MI 48109
Email: tdjtdj@umich.edu
Office: 734-936-1007
For media inquiries: sph.media@umich.edu
Areas of Expertise: Biostatistics